Pest identification and monitoring are essential components in an IPM program. In industrial food manufacturing facilities, pest management professionals monitor insect numbers with glue traps using UV- attraction or sex pheromone. Each trap will be collected and closely examined to identify and count the insects. Identifying and counting the insects is a time-consuming and labor-intensive task, especially when large numbers of glue traps are involved. The identification and counting accuracy could vary between different individuals who must first be trained in insect identification. This study describes two automated insect identification and monitoring devices based on deep-learning technology, named Pest-Vision S (PV-S) and Pest-Vision F (PV-F). Both devices use high-resolution cameras. PV-S scans a glue trap, uploads the image to the cloud for analysis, and the identification and the trapped insect numbers are available in 4 min. On the contrary, PV-F is an on-site device that provides real-time monitoring using IoT. The device takes a glue trap photo every hour and uploads the image to the cloud for analysis. The outcome of the analysis is accessible online, and whenever the insect number exceeds a set threshold, an alert email will be sent. Our study compared the accuracy between manual identification and those by PV-S/PV-F on 25 insect taxa/groups found on glue traps in food facilities. Depending on the prevalence of each taxon/group, the number of verifications ranged from 38–9167. The devices showed highest accuracy (93–95%) when identifying Psychodidae, Phoridae, Drosophilidae, Chironomidae, Dermastidae, Psocodea (winged), and Mycetophilidae, while lowest accuracy was recorded on Scatopsidae (67%) and Ceratopogonidae (69%). Using both devices, the man-hours spent identifying and counting insects on glue traps was reduced by 77.2%. The real-time monitoring could also intercept the infestation at the earliest stage and minimize the number of site visits
during the low insect season.
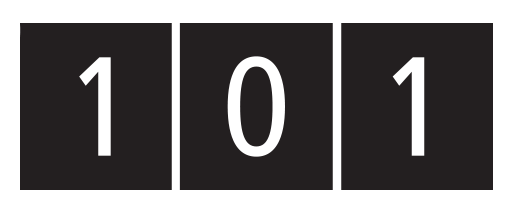
Use Of Deep-Learning Technology For Automated Identification And Monitoring Of Flying Insects In Industrial Food Facilities
Year: 2022
Keywords: iot, remote insect monitoring, ai, object detection
Abstract:
Full Paper